New Scientific Field for Modelling Complex Dynamical Systems: The Cybernetics Artificial Intelligence (CAI)
Cybernetic Artificial Intelligence – A New Paradigm in Modeling Complex Systems
As humanity faces unprecedented challenges in global health, environment, and technology, Artificial Intelligence (AI) has emerged as a powerful tool for problem-solving. Yet, traditional AI approaches often struggle with the intricacies of causality and decision-making in complex dynamic systems. Enter Cybernetic Artificial Intelligence (CAI), a revolutionary interdisciplinary framework that combines principles of cybernetics and AI to model and control complex systems. This blog explores the concepts and implications of CAI as presented in “A New Scientific Field for Modeling Complex Dynamical Systems: Cybernetic Artificial Intelligence (CAI)” and its associated DOI reference.
What is Cybernetic Artificial Intelligence (CAI)?
CAI integrates cybernetic principles—feedback loops, control mechanisms, and system stability—with AI’s computational power. Unlike traditional AI, which often focuses on data-driven predictions, CAI emphasizes understanding the causality behind complex interactions, enabling adaptive and ethical decision-making.
Key Features of CAI:
- Causal Understanding: Differentiates between correlation and causation to improve decision-making.
- Feedback Control: Uses feedback loops for real-time adaptation and optimization.
- Fuzzy Cognitive Maps (FCMs): Combines fuzzy logic and neural networks to model uncertainties and dynamic relationships.
Why Do We Need CAI?
The modern world’s challenges—climate change, pandemics, and geopolitical instability—require holistic approaches that consider interdependencies across systems. Traditional AI often fails to address these complexities, relying heavily on correlations rather than causative analysis. CAI bridges this gap by:
- Modeling complex dynamic systems with causal insights.
- Enabling ethical and human-centric technological development.
- Supporting adaptive systems that can respond to real-time changes.
Historical Roots of CAI
CAI builds on the foundational work of Norbert Wiener, the father of cybernetics, and integrates advancements in fuzzy logic, neural networks, and machine learning. It revives and modernizes the cybernetics framework, which was overshadowed by AI’s rapid rise since the 1950s.
The Cybernetics-AI Divide:
- Cybernetics: Focused on control and communication in systems.
- AI: Initially pursued human-like intelligence, often ignoring cybernetic feedback principles.
CAI unites these two fields, offering a robust approach to address real-world problems.
Applications of Cybernetic Artificial Intelligence
1. Health and Medicine
CAI models have been applied to optimize healthcare systems, predict disease outbreaks, and design personalized treatment plans. For example:
- Fuzzy Cognitive Maps (FCMs) are used to simulate disease progression and evaluate treatment efficacy.
2. Climate and Environment
By incorporating feedback loops and causal analysis, CAI enables better prediction and mitigation strategies for climate change.
- Applications include energy optimization and sustainable resource management.
3. Industry 6.0
CAI supports Industry 6.0, where human-centered technologies collaborate with machines for ethical and efficient outcomes.
- Example: Autonomous systems that adapt to factory workflows while ensuring worker safety.
Fuzzy Cognitive Maps (FCMs): The Backbone of CAI
FCMs are a core component of CAI, combining the strengths of fuzzy logic and neural networks to handle uncertainty and complexity.
How FCMs Work:
- Represent concepts (e.g., variables) as nodes.
- Show causal relationships between nodes with weighted connections.
- Use recursive equations to simulate system behavior over time.
FCMs have been successfully applied to:
- Energy systems optimization.
- Economic forecasting.
- Social dynamics modeling.
Challenges and Ethical Considerations
1. Data Ethics
- Ensuring data used in CAI respects privacy and fairness.
- Avoiding biases that can distort causal models.
2. Transparency and Explainability
- Providing clear explanations for CAI-driven decisions to build trust.
3. Avoiding a New “AI Winter”
CAI addresses the risk of overhyped expectations that could lead to another AI stagnation. Its focus on causality and practical applications ensures sustainable progress.
Conclusion
Cybernetic Artificial Intelligence represents a bold step forward in modeling and solving complex dynamic systems. By integrating cybernetic feedback principles with AI’s computational power, CAI offers a versatile and ethical framework to tackle the world’s most pressing challenges. As we navigate an era of rapid technological advancements, CAI could become the cornerstone of a more sustainable and human-centered future.
Tags
- Cybernetic Artificial Intelligence
- Complex Systems
- Artificial Intelligence
- Fuzzy Cognitive Maps
- Ethical AI
- Industry 6.0
- Dynamic Systems Modeling
- Norbert Wiener
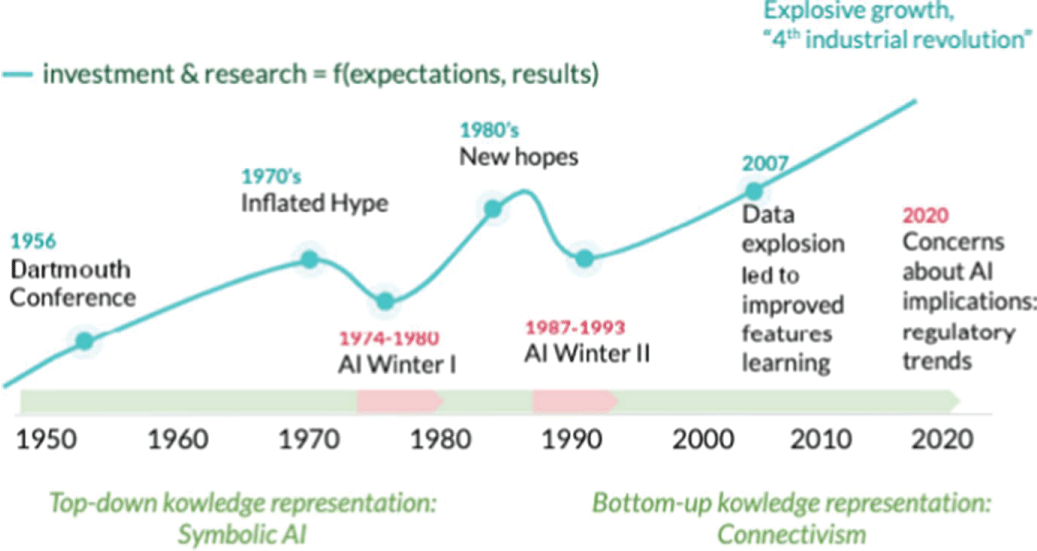
Figure 1: The AI evolution over time
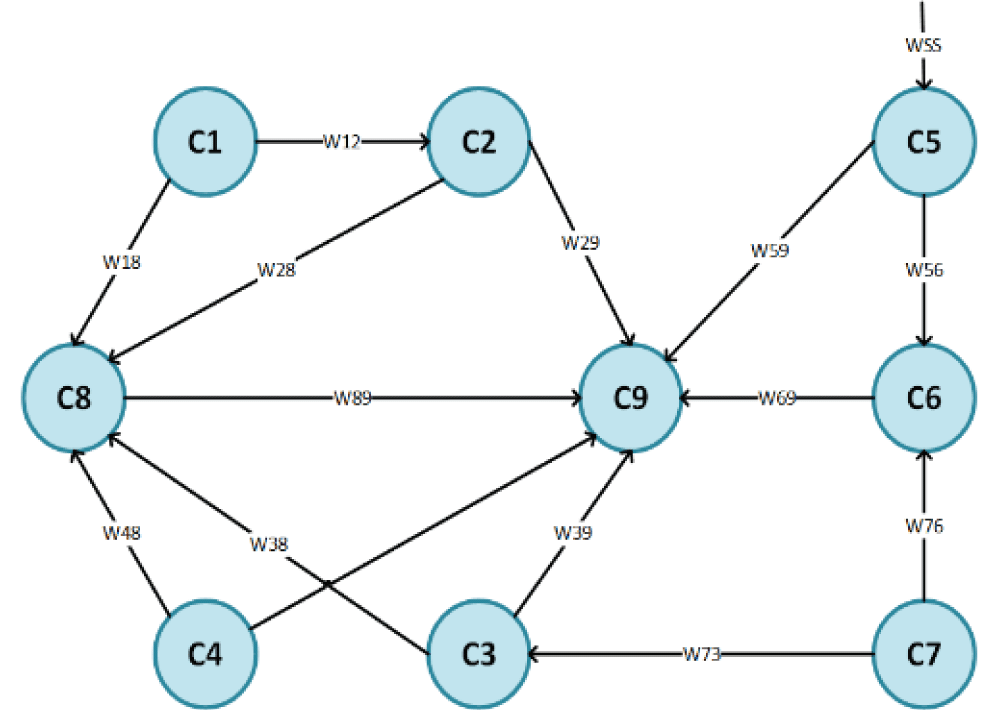
Figure 2: A simple Fuzzy Cognitive Map (FCM)